Was the training data generated using GPT-4o? The quality is questionable.
We know that the three major challenges faced by large models are algorithms, computing power, and data. The first two can be improved through optimization and upgrades, while the latter relies on accumulation. As technology continues to develop, high-quality data has gradually become the biggest bottleneck.
In many new models, to enhance the model's capabilities, people have adopted the method of using AI to generate data for training. It is widely believed that using synthetic data can significantly improve the quality of the model.
However, the latest research suggests that using data generated by AI is not a good approach and may even lead to model collapse.
Today's cover study published in the academic top journal "Nature" believes that if large models are allowed to train themselves with automatically generated data, AI may degenerate and iterate the original content into irreparable nonsense within just a few generations.The research submitted by institutions such as the University of Oxford emphasizes the risk of artificial intelligence model collapse due to self-training, arguing the necessity of original data sources and careful data filtering.
Advertisement
Link to the paper:
Which models are prone to collapse?
The study suggests that irreversible model collapse occurs when artificial intelligence models are overtrained on generated data.
"Model collapse refers to the phenomenon where the model collapses due to indiscriminate training on synthetic data," said Ilia Shumailov, a researcher at the University of Oxford and the lead author of the paper.According to the paper, generative AI tools such as large language models may overlook certain parts of the training dataset, leading the model to be trained only on a portion of the data.
It is well known that large language models (LLMs) require a massive amount of data for training, thereby enabling them to interpret the information within and apply it to various use cases. LLMs are typically constructed to understand and generate text, but the research team found that ignoring the large amount of text it is supposedly reading and incorporating into its knowledge base can quickly turn an LLM into a hollow shell.
"In the early stages of model collapse, the model first loses variance, with performance on a small amount of data deteriorating, and in the later stages of model collapse, the model will completely collapse," said Shumailov. Therefore, as the model continues to train on increasingly inaccurate and irrelevant text generated by the model itself, this recursive loop leads to model degeneration.
What exactly is model collapse?
In this paper, the model collapse effect discovered by the authors is a process of degeneration where the data generated by the model contaminates the training set of the next generation of models. The model, trained on contaminated data, perceives reality incorrectly, as shown in the figure below (a).Model collapse can be divided into early and late stages. Early-stage models will exhibit a decline in performance on a small amount of data, while late-stage models will converge to a distribution that bears little resemblance to the original distribution, and the variance is usually greatly reduced.
Model collapse occurs mainly due to the compounding of the following three specific error sources over several generations of models, leading to significant deviations from the original model:
1. Statistical approximation error. This is the main error caused by the limited number of samples and disappears as the number of samples tends to infinity. This happens because information may be lost at every step of resampling.
2. Functional expression error. This is the second type of error, caused by the limited expressive power of the function approximator. In particular, neural networks are just universal approximators and cannot perfectly approximate any distribution. Neural networks can introduce non-zero likelihood outside the original distribution or zero likelihood within the original distribution. A simple example of functional expression error is if we try to fit a mixture of two Gaussians with a single Gaussian. Even if we have perfect information about the data distribution (i.e., an infinite number of samples), model error will be inevitable. However, in the absence of the other two types of errors, this situation can only occur in the first generation of models.
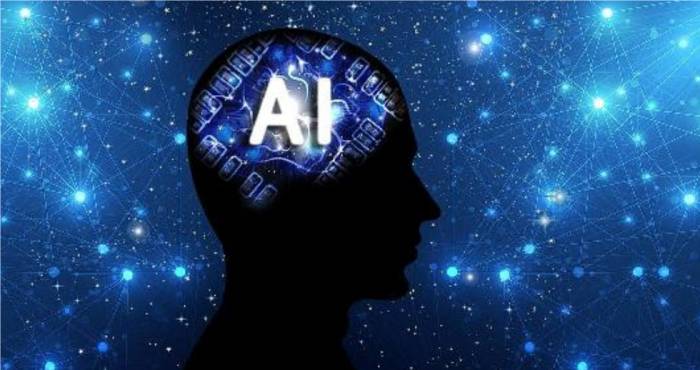
3. Function approximation error. This is a secondary type of error, mainly stemming from the limitations of the learning process, such as the structural bias of stochastic gradient descent.The above factors can each lead to model collapse becoming worse or better. Higher approximation capability can even be a double-edged sword; better expressiveness can offset statistical noise, thus closely approximating the true distribution, but it can also amplify noise. This often results in a cascading effect, where inaccuracies of individuals combine to cause an increase in overall error.
For instance, overfitting to the density model can lead to incorrect inferences by the model, assigning high-density areas to low-density areas not covered by the training set.
It is worth noting that there are other types of errors as well. For example, the precision of computers is limited in practice.
Model Collapse in Language Models
The authors also assess the impact of model collapse on language models in the text. Model collapse is prevalent in various machine learning models. However, unlike small models that are usually trained from scratch (such as GMMs and VAEs), Large Language Models (LLMs) require a huge cost to train from the beginning, so they are often initialized using pre-trained models (such as BERT, RoBERTa, or GPT-2), which are trained on large text corpora. Subsequently, these models are fine-tuned to adapt to various downstream tasks.In this paper, the author explores what happens when language models are continuously fine-tuned using data generated by other models. All experiments discussed in this paper can be easily replicated in a non-fine-tuning setting with larger language models. Given that training a medium-sized model also requires considerable computational power, the author chose not to conduct such experiments, but instead focused on more realistic proof-of-concept settings.
It should be noted that the language experiments described in this paper still take several weeks to complete even under these circumstances. The author evaluated the most common setting for training language models — the fine-tuning setting, where each training cycle starts from a pre-trained model with the latest data. Here, the data comes from another pre-trained model that has been fine-tuned. Since the training is limited to generating models that are very similar to the original pre-trained model, and the data points generated by these models usually produce only very small gradients, it is expected that the model will only undergo moderate changes after fine-tuning. The author fine-tuned the OPT-125m causal language model provided by Meta through Hugging Face.
Case Study: Churches and Jackrabbits
The researchers provided an example in the paper using the text generation model OPT-125m (fine-tuned with the wikitext2 dataset), which performs similarly to ChatGPT's GPT-3 but requires less computational power.
The researchers input text about the design of 14th-century church towers into the model. In the first generation of text output, the model mainly discussed buildings constructed under the reign of different popes. But by the ninth generation of text output, the model mainly discussed a large number of black-tailed, white-tailed, blue-tailed, red-tailed, and yellow-tailed jackrabbits. What we should note is that most of these are not real species of jackrabbits.The content output by the large model: From the church to over 100 languages, and then to wild rabbits.
The experimental results show that even if the original data is always retained, the phenomenon of model collapse can still occur. As the iterations continue, the model begins to forget the information in the real data, and the content it generates contains more and more repetitive phrases.
The internet is flooded with AI content, and the "data source" has long been contaminated.
You might ask at this point: Isn't it simple, just don't train AI with synthetic data? But in fact, the "data" we can obtain from the internet now, it is unknown how much of it is generated by AI, and we often cannot distinguish it from normal content.
It is not new that the internet is filled with various content. As researchers pointed out in the paper, before large-scale language models (LLMs) became a well-known topic to the public, malicious websites had already been creating content to deceive search algorithms to prioritize their websites to get clicks. With the advent of OpenAI's GPT series of large models, generative AI has and will greatly change the ecology of text and image content.AI-generated text can be produced much faster than humans can speak nonsense, which has sparked larger-scale concerns. Emily Wenger, a computer scientist at Duke University who specializes in privacy and security, once wrote about this in an article: "Although the impact of AI-generated content on the internet on humans is yet to be observed, Shumailov and others reported that the proliferation of AI-generated content online could have a devastating effect on the models themselves."
"One of the problems brought about by model collapse is the challenge it poses to the fairness of generative AI. Collapsed models will overlook some of the less common elements in the training data, thus failing to reflect the complexity and nuances of the world," Wenger added, "This could lead to a reduction in the representation of minority groups or viewpoints, and they may even be erased."
Large technology companies are taking some measures to reduce the amount of AI-generated content seen by ordinary internet users. In March, Google announced that it would adjust its algorithms to lower the priority of pages that appear to be designed for search engines rather than human searchers. However, this statement was released after 404 Media's report on Google News promoting AI-generated articles.
The study featured on the cover of "Nature" magazine emphasizes that accessing raw data sources and carefully filtering data in recursively trained models helps to maintain the accuracy of the models.
The study also suggests that the AI community creating large language models (LLMs) can coordinate and collaborate to track the sources of information input into the models. "Otherwise, as this technology becomes widely used, if it is not possible to obtain data crawled from the internet before the technology becomes widespread or a large amount of human-generated data, training new versions of LLMs may become increasingly difficult," the research team concluded.