Will human doctors be out of a job due to large models like ChatGPT?
This concern is not unfounded. After all, Google's large model (Med-PaLM 2) has easily passed the United States Medical Licensing Examination, reaching the level of medical experts.
However, a recent study suggests that in clinical aspects, human doctors outperform current artificial intelligence (AI) models, so there is no need to worry too much about personal "unemployment issues."
The relevant research paper, titled "Evaluation and mitigation of the limitations of large language models in clinical decision-making," was recently published in the scientific journal Nature Medicine.
The study found that even the most advanced large language models (LLMs) currently cannot make accurate diagnoses for all patients, and their performance is significantly worse than that of human doctors—
The diagnostic accuracy rate of doctors is 89%, while the diagnostic accuracy rate of LLMs is only 73%. In an extreme case (diagnosis of cholecystitis), the LLM's accuracy rate is only 13%.
What is even more surprising is that as the LLMs gain more knowledge of the case information, their diagnostic accuracy will decrease instead, and sometimes they will even request some examinations that may pose serious health risks to the patients.
Advertisement
How does LLM perform as an emergency department doctor?
Although LLM can easily pass the United States Medical Licensing Examination, the medical licensing examination and clinical case challenges are only suitable for testing the examinee's general medical knowledge, which is far less difficult than the daily complex clinical decision-making tasks.
Clinical decision-making is a multi-step process that requires collecting and integrating data from different sources and continuously assessing facts to reach evidence-based patient diagnosis and treatment decisions.To further explore the potential of Large Language Models (LLMs) in clinical diagnosis, a research team from the Technical University of Munich and its collaborators created a dataset based on the Medical Information Mart for Intensive Care (MIMIC-IV) database. The dataset encompasses 2400 real patient cases and four common abdominal conditions (appendicitis, pancreatitis, cholecystitis, and diverticulitis), simulating a realistic clinical environment that replicates the process from emergency to treatment, thereby assessing its applicability as a clinical decision-maker.
Figure | Data source and evaluation framework. The dataset originates from real cases in the MIMIC-IV database, containing comprehensive electronic health record data recorded during hospitalization. The evaluation framework reflects a realistic clinical environment, conducting a comprehensive assessment of LLMs based on multiple criteria, including diagnostic accuracy, adherence to diagnostic and treatment guidelines, consistency in following instructions, the ability to interpret laboratory results, and robustness to changes in instruction, information volume, and information sequence. ICD, International Classification of Diseases; CT, Computed Tomography; US, Ultrasound; MRCP, Magnetic Resonance Cholangiopancreatography.
The research team tested Llama 2 and its derivatives, including general versions (such as Llama 2 Chat, Open Assistant, WizardLM) and models aligned with the medical field (such as Clinical Camel and Meditron).
Due to privacy issues with MIMIC data and data usage protocols, the data could not be used for external APIs such as OpenAI or Google, thus preventing the testing of ChatGPT, GPT-4, and Med-PaLM. It is worth noting that the performance of Llama 2, Clinical Camel, and Meditron in medical licensing exams and biomedical question-answering tests has reached or even surpassed that of ChatGPT.
The control group consisted of four internists from two countries with varying years of emergency experience (2, 3, 4, and 29 years, respectively). The results showed that the performance of LLMs in clinical diagnosis was far inferior to that of human doctors.
1. The diagnostic performance of LLMs is significantly lower than that of clinical doctors. The results showed that the overall performance of current LLMs is significantly inferior to that of doctors (P < 0.001), with a diagnostic accuracy gap ranging from 16% to 25%. Although the model performed well in the diagnosis of simple appendicitis, it performed poorly in the diagnosis of other pathologies such as cholecystitis, especially the Meditron model, which often misdiagnosed patients with "gallstones" in cholecystitis.
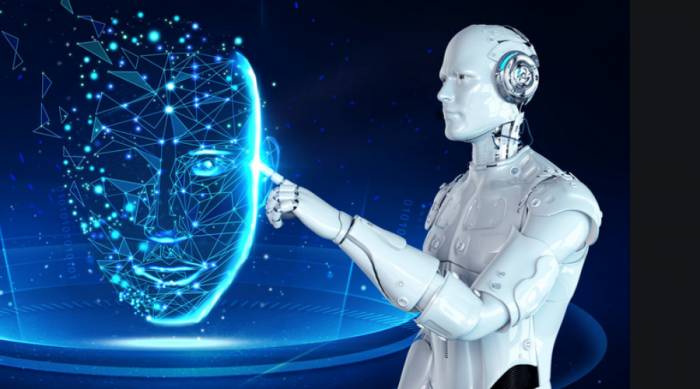
Specialized medical LLMs did not show a significant advantage over other models in overall performance, and their performance further declined when LLMs needed to collect all information on their own.2. Clinical Decision-Making of LLMs is Hasty and Unsafe
The research team found that LLMs perform poorly in following diagnostic guidelines, easily omitting important physical information of patients. In addition, there is a lack of consistency in arranging necessary laboratory tests for patients. LLMs also have obvious deficiencies in interpreting laboratory results. This indicates that they make hasty diagnoses without fully understanding the patient's case, posing a serious risk to patient health.
3. LLMs Still Require Extensive Clinical Supervision by Physicians
Furthermore, all current LLMs perform poorly in following basic medical guidelines, making errors in 2-4 out of every 2-4 cases, and fabricating non-existent guidelines in 2-5 out of every 2-5 cases.
Performance of LLMs under Different Data Volumes. The study compared the performance of each model using all diagnostic information versus using only a single diagnostic test and the current medical history. For almost all diseases, in the MIMIC-CDM-FI dataset, providing all information did not lead to the best performance. This indicates that LLMs cannot focus on key facts, and their performance will decline when too much information is provided.
The study also shows that the order of information that provides the best performance for each model is different for each pathology, which undoubtedly further increases the difficulty of optimizing models in the future. Without extensive physician supervision and prior assessment, tasks cannot be reliably completed. Overall, they have obvious defects in following instructions, processing information order, and handling relevant information, thus requiring a large amount of clinical supervision to ensure their correct operation.
Although the study found various problems of LLMs in clinical diagnosis, the prospects of LLMs in the medical field are still huge, and they are more likely to be more suitable for making diagnoses based on medical history and test results. The research team believes that there is room for further expansion in the following two aspects of this research work:
Model Validation and Testing: Further research should focus on more comprehensive validation and testing of LLMs to ensure their effectiveness in real clinical environments.Interdisciplinary Collaboration: It is suggested that AI experts work closely with clinicians to jointly develop and optimize large language models (LLMs) suitable for clinical practice and address issues in practical application.
How is AI Disrupting Medicine?
Not only the aforementioned research, but also a team from the National Institutes of Health (NIH) and its collaborators have discovered similar issues—when answering 207 image challenge questions, GPT-4V scored high in selecting the correct diagnosis, but often made mistakes in describing medical images and explaining the reasons behind the diagnosis.
Although AI is still far from being as proficient as human professional doctors, its research and application in the medical industry has always been an important "battlefield" for competition among technology companies and research universities at home and abroad.
For example, Google released the medical AI large model Med-PaLM2, which has powerful diagnostic and treatment capabilities, and is also the first large model to reach the "expert" level in the MedQA test set.
The "Agent Hospital" proposed by the research team from Tsinghua University can simulate the entire process of treating diseases. Its core goal is to enable doctor agents to learn how to treat diseases in a simulated environment, and even continuously accumulate experience from successful and failed cases to achieve self-evolution.
Harvard Medical School led the development of a visual language general AI assistant for human pathology—PathChat, which can correctly identify diseases from biopsy sections in nearly 90% of cases, outperforming current general AI models and professional medical models such as GPT-4V.
Recently, OpenAI CEO Sam Altman co-founded a new company, Thrive AI Health, aimed at helping people improve daily habits and reduce the mortality rate of chronic diseases with the help of AI technology.
They claim that ultra-personalized AI technology can effectively improve people's living habits, thereby preventing and managing chronic diseases, reducing the medical economic burden, and improving people's overall health level.Today, the application of AI in the medical industry has gradually transitioned from the initial experimental phase to the practical application phase. However, there may still be a long way to go before it can help clinical doctors enhance their abilities, improve clinical decision-making, and even directly replace them.