Nonsense is not to be feared; it is the nonsense spoken with a straight face that is truly terrifying. What's even more terrifying is that you believe in someone's nonsense because they speak with a straight face... This is the current reality we must face when using AI (holding our noses).
How can we avoid AI generating false factual content that misleads users? Various large model platforms have been researching and trying to address this issue. To "avoid" the problem, we must first "identify" the problem. On June 19, a research team from the University of Oxford published a new study in the journal Nature, proposing a promising method for "lying detection" in AI. Let's discuss this in detail.
The nonsense and risks of large models
"Hallucinations" are a key issue faced by large language models (such as ChatGPT, Gemini, or Wenxin Yiyan), and are also one of the common types of user experience complaints on the internet. This term can be roughly understood as AI speaking nonsense with a straight face.
For example, if you ask ChatGPT: What does it mean for a dinosaur to carry a wolf?
It will tell you with a straight face - this symbolizes the confrontation between old and new forces, a game between a weak but clever and flexible challenger and a powerful but inflexible opponent.
Advertisement
The answer is very soul-cleansing, rising to the level of philosophy and values, but it is nonsense.
Click to enter a picture description (up to 30 words)
This is just one of the common types of "hallucinations" in large language models, and other types include:1
Erroneous Historical Facts
"Who was the first president of the United States?" ChatGPT responded: "Thomas Jefferson."
2
Incorrect Scientific Information
"What is the boiling point of water?" ChatGPT responded: "The boiling point of water at standard atmospheric pressure is 120 degrees Celsius."
3
Fabricated Quotes, AI Frankenstein
"What did Einstein say about relativity?" ChatGPT responded: "Einstein once said in the book 'Relativity and Reality,' 'Time is an illusion.'" Although Einstein did discuss the relativity of time, he did not make this statement in the so-called book 'Relativity and Reality.' In fact, this book may not even exist. It is a fabricated quote by the model.
4
(The original text ends abruptly here, so there is no content to translate for point 4.)Misleading Health, Legal, and Financial Advice
You ask, "What medicine should I take for a cold?" ChatGPT replies, "You should take antibiotics for a cold."
In addition to the above question, I believe everyone will also encounter other nonsensical situations when using AI. Although various large models are actively dealing with these issues, many of the examples mentioned above may have been fixed, but it has always been difficult to find a "radical cure" or "elimination" method for these problems. In terms of testing and judgment, it often requires manual feedback or data set annotation, which brings a considerable cost.
This greatly reduces our experience of using AI - who dares to trust an assistant who talks nonsense without reservation? Moreover, some issues are related to health and safety, and making mistakes can cause big problems.
Is there any way to more universally "calculate" whether AI is talking nonsense?
How can "semantic entropy" help large models detect lies?
Recently (June 19), a team from the University of Oxford published a paper in the journal "Nature," proposing a new analysis and calculation method, opening up new ideas for solving the "hallucination" problem of large language models.
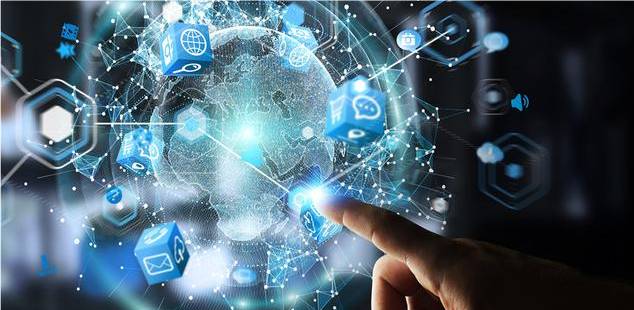
Click to enter a picture description (up to 30 characters)
The team proposed a statistical entropy estimation method called "semantic entropy" to detect "fabrication" in large language models, that is, the "nonsense syndrome" that large models have been criticized for. The authors tested the semantic entropy method on multiple datasets, and the results show that the semantic entropy method is significantly better than other benchmark methods in detecting fabrication.So, what exactly is "semantic entropy"?
Setting aside lengthy professional explanations, we can simply understand semantic entropy as an indicator of probability statistics, used to measure whether the information in a set of answers is consistent. If the entropy value is low, meaning everyone gives similar answers, it indicates that the information is credible. However, if the entropy value is high, and the answers are all different, it suggests that there might be an issue with the information.
This is somewhat similar to the idea that if a person is lying, they may not be able to fabricate the details of the lie exactly the same each time. A lie often requires countless other lies to support it. From an information theory perspective, this may introduce more uncertainty and randomness. The liar needs to introduce additional information or details to support their false narrative, which may increase the uncertainty or entropy of the information, and thus be detected by algorithms.
For example, when you ask an AI, "What is the highest mountain in the world?"
A large model might give several answers: "Mount Everest," "Mount Kilimanjaro," "Andes."
By calculating the semantic entropy of these answers, it is found that the answer "Mount Everest" appears most frequently, while other answers rarely or never appear. A low semantic entropy value indicates that "Mount Everest" is a credible answer.
Semantic entropy has both advantages and weaknesses.
The advantage of the semantic entropy detection method is that it does not require any prior knowledge, nor does it need additional supervision or reinforcement learning. In layman's terms, when using this method, you don't need to know everything about the world; you just need to see what everyone else says when you're unsure.
However, currently common methods such as labeled data and adversarial training do not have as good "generalization" effects (i.e., the ability to draw inferences from one instance to another) as calculating semantic entropy. Even in new semantic scenarios that large models have never encountered, the semantic entropy method can be applied.
Of course, although semantic entropy is a relatively effective method, it is not a panacea, and it has its own limitations:Limited ability to handle ambiguity and complexity
Semantic entropy may not be effective enough when dealing with very ambiguous or complex issues.
When faced with questions that have multiple possible correct answers, such as "What is the best programming language?", semantic entropy may not be able to clearly distinguish which answer is more reliable, as multiple answers could be reasonable.
(Who says it's Python? I C++ the first to disagree!!)
Ignoring context and common sense
Semantic entropy is mainly based on statistical and probabilistic calculations, which may overlook the impact of context and common sense. In some issues that require a comprehensive judgment of context and common sense, semantic entropy may not provide an accurate reliability assessment. For example, friends who often fall in love may have experienced this: between lovers, a sentence: "I'm fine, you go ahead and busy."
Do you think TA is really fine, or is there a big problem?
In this case, it is necessary to judge based on contextual scenes, character states, and other information, and different contexts will lead to different understandings. Semantic entropy can only evaluate based on the statistical probability of words, which may give the wrong judgment.For instance, consider common-sense judgments, which are the objective laws of the physical world. Suppose we ask a question: "From which side does the sun rise?"
The correct answer is "the east." However, if we have the following two candidate answers:
1. The sun rises from the east.
2. The sun rises from the west.
(This may be due to biases in the model's training data and the randomness of the generation process)
Even if semantic entropy detects that the probability distribution of the two answers is close, common sense tells us that answer 1 is the correct one. Semantic entropy may not provide enough information to judge the reliability of the answer in this case.
3
If the training data is unintentionally or deliberately "contaminated," semantic entropy also cannot identify it well.
If incorrect data is used to imprint "ideological steel marks" on large models, the model is very "confident" in its generation of incorrect statements (i.e., incorrect statements dominate in the model's output probability distribution), then the entropy value of these statements may not be very high.
In summary, from the perspective of the content generation mechanism of large models, the "illusion" problem cannot be completely avoided. When using AI-generated content, it is best to manually verify important mathematical reasoning, historical events or scientific conclusions, legal and health knowledge, and other aspects.However, from another perspective, "illusions" are also a manifestation of the creativity of large language models, and we may want to make good use of the "illusion" capabilities of large models. After all, illusions are not necessarily bugs (malfunctions), but rather features (characteristics) of large models.
If we need to retrieve facts, we already have search engine tools. But if we need someone to help us edit a nonsensical script like "a dinosaur carrying a wolf," then a large language model is obviously a better assistant.
Click to enter a description of the image (up to 30 characters)
For example, the author racked his brains to draw a picture of a dinosaur carrying a wolf, but a certain AI was impervious to reason and drew a picture of a dinosaur seemingly swallowing a wolf, no wonder it couldn't understand the true meaning of a dinosaur carrying a wolf...